Deep fakes have the power to change the world—both for the good and the bad. They can damage somebody’s reputation or stimulate international political debates for the sake of the planet. Its uses are what matters. Let’s learn what deep fake technology is and how it can serve businesses and the world.
Deep Fakes: How They Work (Or Network Like a Pro)
A deep fake is a video, photo, or audio recording that appears real but has been manipulated with artificial intelligence. The technology can replace faces, manipulate facial expressions, and synthesize speech.
Regardless of the particular media type, each deep fake comes to life through three stages typical of all AI-based products. These are: data extraction and processing, ML-model training, and conversion. It all sounds quite techy, so let’s illustrate these transformations with an example.
John and Jane are siblings working in competing digital transformation agencies. They are going to participate in a virtual digital transformation exhibition. Their goal is to make as many useful business contacts as possible. Jane thinks her brother is in a better position because he is a man. John, in turn, thinks his sister has a better chance because she is a woman. Since the exhibition takes place online, they decide to virtually swap their faces and voices.To do this, they use deep fake technology. Let’s see an example of what the process of video deep fake creation looks like.
Step 1: Extraction
The alignment is critical, because the neural network that performs the face swapping requires all faces to be the same size and have all features aligned. It’s also crucial to use high quality images in the input, since it will define the quality of the output.
Step 2: Training
At the training stage, a neural network will learn to convert John’s face into Jane’s and Jane’s into John’s. This step is commonly performed using a specific deep network architecture known as an autoencoder. It consists of three parts:
- An encoder recognizes features of an input face
- A latent space reduces a face to its basic features
- A decoder reconstructs the input face with all details
Autoencoding has to be repeated for each face. This means we need two autoencoder networks—one for John and one for Jane. At first, the networks learn to recognize facial features, then reduce the face to its basic features, and then reconstruct the face again.
Then, the networks are trained with hundreds or even thousands of facial expressions of each sibling. Usually, the training process takes hours. At the beginning, the results will still be subpar, and the generated faces will be barely recognizable. But by constantly trying and failing, the network will learn to restore a face more realistically.
When the networks are trained enough, the decoders will be swapped. John’s facial expressions will be generated with Jane’s decoder instead of his own. In the process, his facial gestures will be retained, but reconstructed with Jane’s face, and vice versa.
This will allow John's network to easily recognize Jone’s facial expressions and reconstruct them with Jane's charming smile. Similarly, Jane’s network will transform her into a self-confident man.
Step 3: Conversion
When the training is complete, the final step is to merge Jane’s converted face back into John’s original frame (and do the same for Jane). It’s also possible to tweak settings that change the coloring of the output, rescale the overlaid image, and more. Then all the converted frames will be merged into a video.
In the end, this idea worked for both John and Jane. They were able to achieve their goals by taking advantage of the opposite sexes. John came across as a pleasant and charismatic young woman. Jane gave the impression of an active, trustworthy man. John and Jane learned there was no benefit to be gained from appearing stereotypically male or female, but the experiment did make them more confident!
How Businesses Can Benefit from Deep Fakes
There’s plenty of potential for deep fake technology to benefit businesses when used within ethical grounds. Here are some real-life examples.
Healthcare
The Canadian AI startup Lyrebird creates custom products using deep fake voice technology. The company is working with the ALS Association on "Project Revoice'' to help people with ALS, or Lou Gehrig's disease. The initiative enables people with ALS who have lost the ability to talk to continue speaking in their own authentic and personal voice.
Marketing
Rosebud AI, a San Francisco-based company, uses deep fake technology to generate modeling photos. They released a collection of 25,000 modeling photos of people that never existed, along with tools that can swap synthetic faces into any photo. Rosebud AI also launched a service that can put clothes photographed on mannequins onto virtual but real-looking models. This way, the company intends to help small brands with limited resources create more powerful image portfolios with more diverse faces.
Exhibitions
The Dalí Museum in St. Petersburg, Florida used deep fake technology as part of their exhibition. A life-sized deep fake of the surrealist artist (1904-1989) appeared before visitors when they pressed the doorbell on the kiosk, and then told visitors stories about his life. The deep fake was created using archival footage from interviews with 6,000 frames. It took 1,000 hours to train the AI algorithm on Dalí’s face.
E-commerce
AI company Tangent.ai has developed a deep fake-based solution for e-commerce. The tool enables shoppers to determine what products will look like on them. For example, a consumer may change a model’s lipstick, hair color, ethnicity, or race. After deploying the platform, Tangent’s clients increased their sign-up conversion rate by up to 64%.
Film Production
Deep fakes can be applied to movie-making as well. The technology can recreate the faces of actors who have passed away so that their character won’t have to die with them. A good example of this is the recreation of Peter Cushing in Star Wars: Rogue One (2017), who passed away in 1994. Using deep fakes, it’s also possible to de-age actors as was the case with Will Smith in Gemini Man.
Deep Fakes: Possible Pitfalls
Deep fake technology is one of the most worrying fruits of rapid advances in artificial intelligence. When misused, it presents multiple ways to damage businesses. For example, competitors can use video and audio clips that appear to be real to ruin the reputations of companies and their executives. Celebrities such as Gal Gadot, Hilary Duff, Emma Watson, and Jennifer Lawrence have already had to confront this when their likenesses were inserted into explicit scenes.
There is also another way to misuse deep fakes. The CEO of a U.K.-based energy company believed he was speaking on the phone with his boss. He asked him to immediately transfer €220,000 (approx. $243,000) to the bank account of a Hungarian supplier. The transferred money was then moved to Mexico and other locations abroad. The company never recovered these funds.
Bottom Line
Today's deep fake technology has not yet reached the level of totally authentic material. In most cases, it’s still possible to distinguish a deep fake from the original with a close look. Even though the technology is gradually getting better, creating a high-quality deep fake, especially in real time, is still no trivial matter. From our experience, deep fake-based products require technical expertise and powerful equipment, like gaming PCs powered by Nvidia's GPUs. Still, deep fake products can change the world for the better and have huge business potential.
More articles on the topic
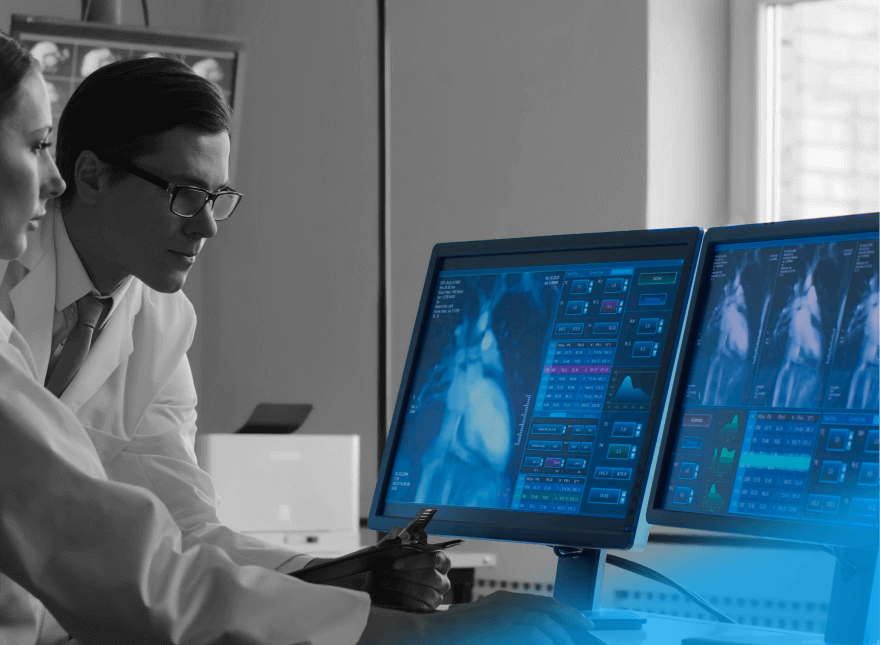
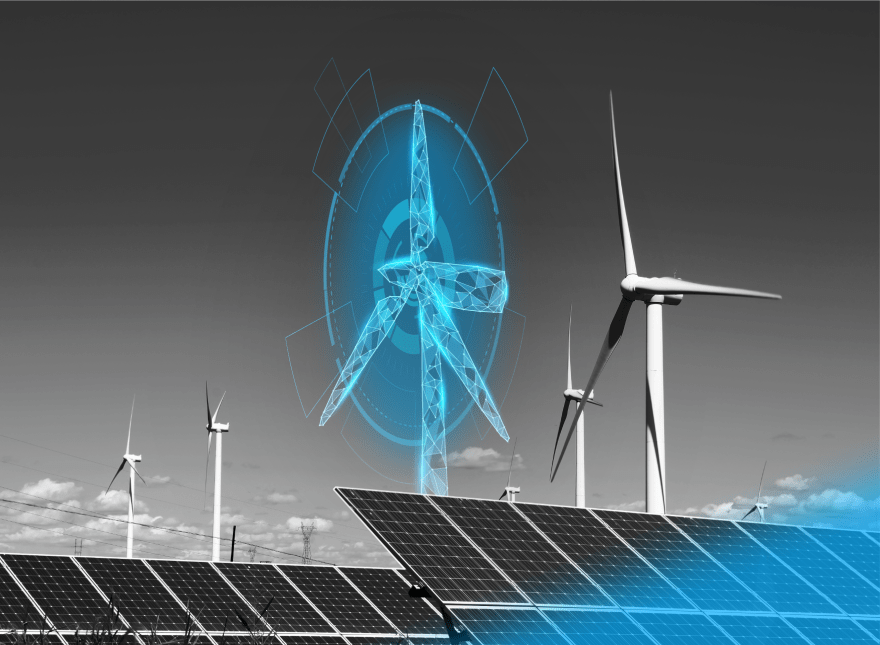
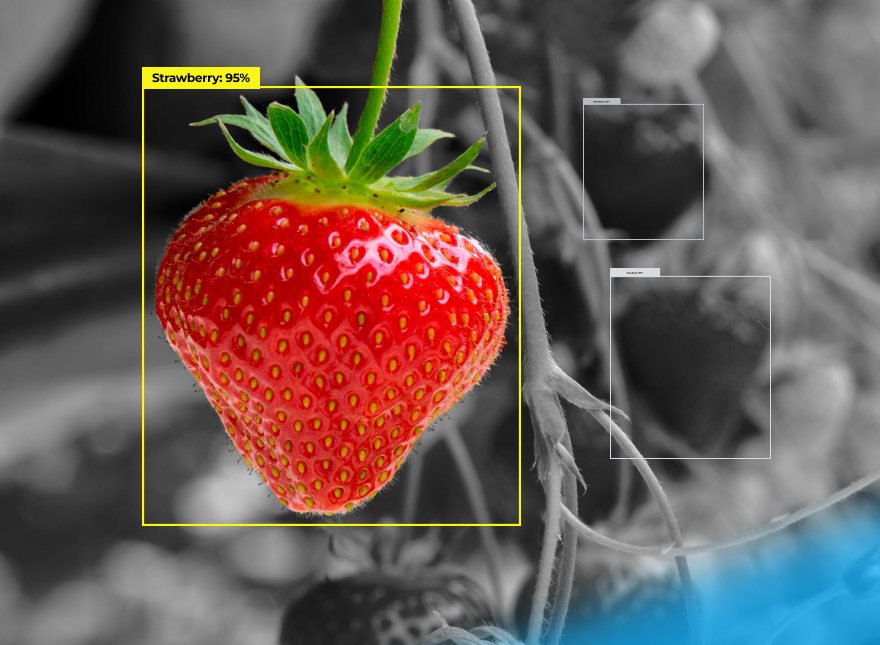
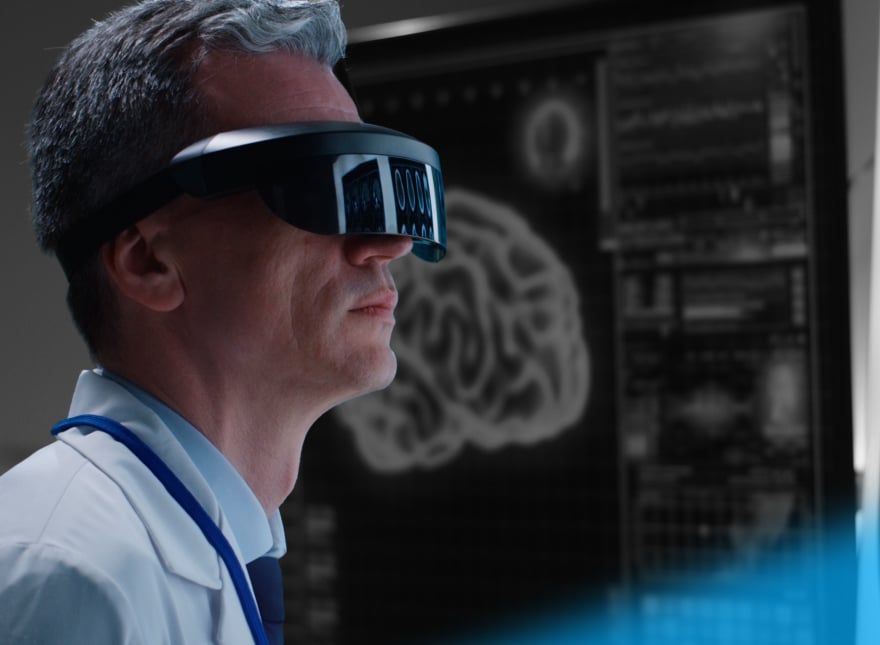
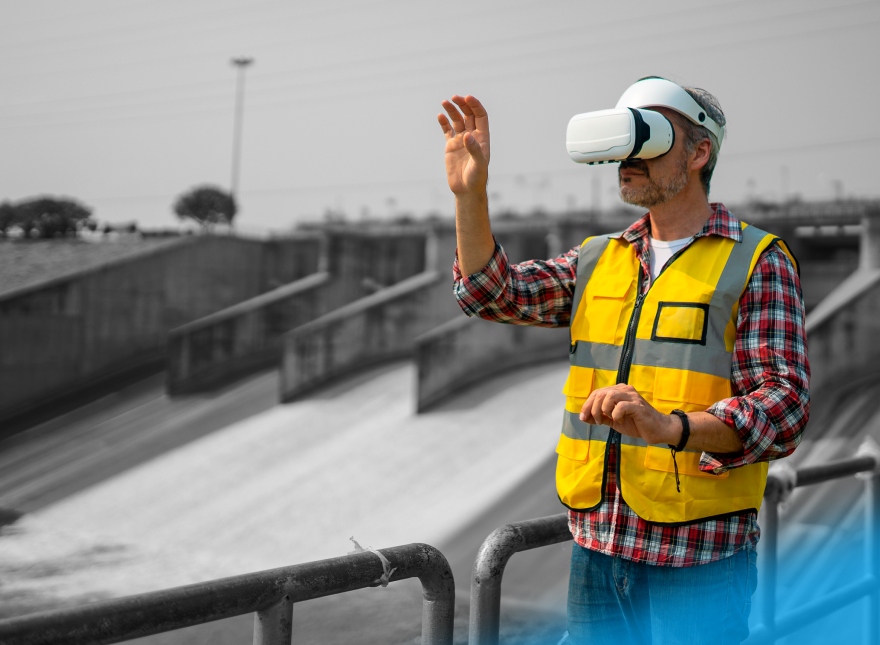
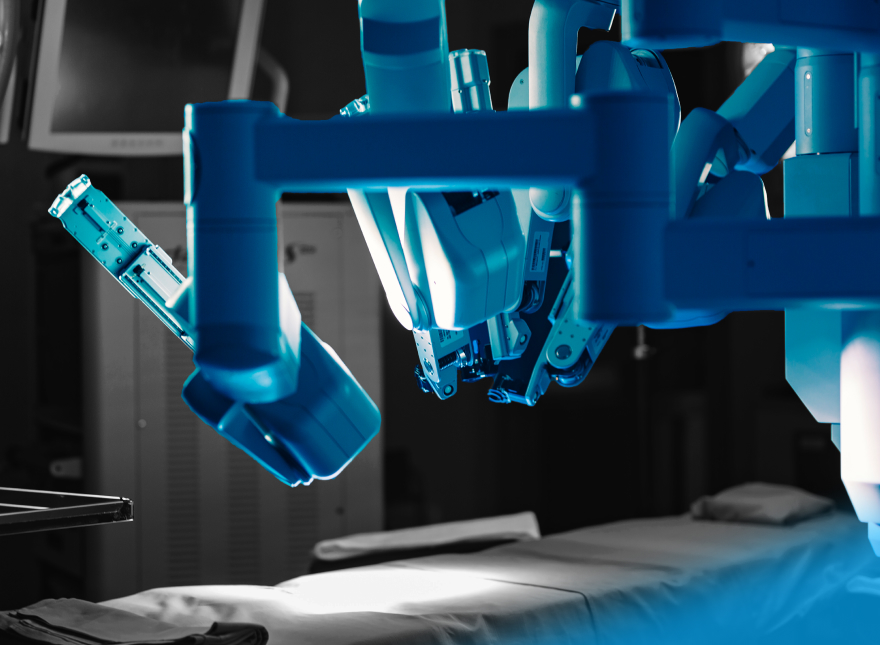