For years, healthcare adopted a one-size-fits-all approach. The main reason for this was a lack of technology to enable affordable personalization. But times have changed. With the surge in healthcare data and the rapid advancement of artificial intelligence and big data, personalized medicine has become a reality.
For tech companies, the combo of big data and AI in personalized medicine has created a pool of opportunities. While big data provides valuable information for precise treatments, AI comes with robust capabilities to process this data. This synergy is a game changer for the healthcare industry.
This article is an enlightening exploration of how AI, combined with big data in healthcare, can be transformative. Check out the promising use cases with real-world examples below.
Key Elements at Play in Precision Medicine
Precision (personalized) medicine is a way for healthcare providers to offer specific care tailored to each individual patient. To achieve this, clinicians don’t just rely on the patient’s medical history and symptoms. They use genetic information, biomarkers, and other physiological data. This enables each patient to receive a custom treatment plan and avoid taking drugs that lead to adverse reactions. Doctors are also less likely to prescribe expensive treatments that turn out to be ineffective.
To personalize care, precision medicine needs access to massive amounts of data and the right tech capabilities to process it. There are two key elements at play:
Data collection and preparation. This is where big data is used. Big data refers to extremely large and complex datasets. To process them, you need sophisticated analytics tools and technologies. These datasets can come from various sources at high speed and need to be processed promptly. Examples include genetic or lifestyle information and medical history. These datasets can be used to select more effective treatments.
Data interpretation. Artificial intelligence comes into play here. AI can process large amounts of real-time data, detect patterns, and generate insights. For example, AI in precision medicine can interpret vast genomic datasets. With machine learning and deep learning techniques, it can see hidden links in these datasets. As a result, clinicians can create genome-informed treatment plans for their patients.
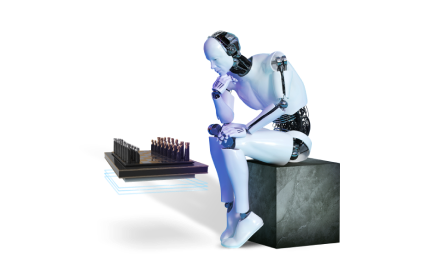
The combo of AI and big data is full of potential for the healthcare sector. At the same time, it’s a prospective niche for companies that build digital solutions for healthcare. Some are already hitting the market with their solutions.
Now, let’s take a look at three common use cases of AI and big data in healthcare with a focus on precision medicine (with real-world examples).
Use Case 1. AI and Big Data in Cancer Research and Treatment
Cancer cases are rising every year around the world and particularly in the US. The estimated number of new US cases climbed from 1.7 million in 2020 to almost 1.96 million in 2023. According to the stats, almost one in three patients will die from cancer. This puts significant pressure on oncologists and health services. The good news is that diagnosing new cases early could cut this number. This is where the combo of AI and big data comes into play.
Big data can unlock valuable insights into cancer biology and risk factors. One such risk factor is specific molecular signatures linked to different cancer types. AI can analyze genomic and molecular data and detect molecular signatures like the BRCA mutation. Experts know that the BRCA mutation increases the risk of breast and ovarian cancers. Women can undergo genetic testing to find out if they have this mutation. If they do, they can receive more regular screening (like mammograms or ultrasounds of the ovaries).
Access to pharmaceutical datasets also accelerates cancer research, and many are now public. One of them is CCLE—an encyclopedia of over 1,000 cancer cell lines with genetic and pharmacologic characteristics. These cancer cell lines are essential for understanding cancer biology, validating cancer targets, and assessing drug efficacy.
Real-Life Example
Oncora Medical has created a patient care platform for oncologists. It integrates electronic health records (EHRs), cancer registries, and other software used in oncology clinics. Radiology and pathology reports and diagnostic tests are all in one tool. It provides doctors with information about each patient’s condition in a structured way. With ML, the solution identifies patients with the highest risk of adverse events.
Moreover, the solution undertakes routine data documentation tasks. This means clinicians can focus on treating patients.
The MD Anderson Cancer Center uses this tool. Since they don’t need to enter the same patient data into different platforms, data documentation takes them 67% less time.
Benefits
- Better understanding of cancer biology and risk factors
- Early detection and diagnosis of cancer
- Custom treatment plans for patients with cancer
- Better patient outcomes
Use Case 2. AI and Big Data in Disease Detection
Whether it’s for cancer or an autoimmune disorder, early detection may result in a better outcome for the patient. But diagnostic testing isn’t always simple or quick. Consider autoimmune diseases. On average, it takes 4.6 years to diagnose a serious autoimmune disease. During that period, a patient typically visits five doctors. Symptoms can flare up and ease off over time, and they vary from one person to another.
The combo of AI and big data helps speed up the diagnosis of autoimmune and other health conditions. Tech advances, like gene sequencing, help identify patients at high risk. Gene sequencing is helpful for diseases that have a genetic predisposition because it identifies changes in certain areas of the genome. This helps scientists understand a disease’s pathogenesis and incidence rate. AI models trained on large genetic datasets can provide these insights.
The technology also offers a new way to treat patients. For example, ribonucleic acid (RNA) data and ML algorithms help describe a patient’s genes. Doctors can use this information to group their patients. This enables them to receive personalized care that suits their specific needs instead of standard treatment.
Real-life Example
PeraHealth, a US-based company, created the Rothman Index, a scoring system for overall patient health. It takes the data from EHRs, vitals, and lab results to assign a score. The tool provides clinicians with a visual dashboard that helps them monitor a patient’s condition in real time. Thanks to ML, doctors can determine if a patient needs their intervention. This ensures clinicians identify at-risk patients and note changes before they become life-threatening.
Using the Rothman Index, doctors can detect diseases early and enable better health outcomes (e.g., fewer patients being transferred to intensive care units). Yale-New Haven Health cut the mortality rate of their patients from sepsis by 29%. Meanwhile, Shannon Skilled Nursing Facility lowered hospital readmission rates by 14%.
Benefits
- Detecting diseases at early stages
- Identifying high-risk patients
- Personalizing treatment plans based on genetic and other data
- Reducing readmission rates
Use Case 3. AI and Big Data in Drug Discovery
New drug discovery is an extremely complex and time-consuming process. It can take more than a decade—from finding a specific target associated with a particular disease to gaining regulatory approval. In addition, the cost of creating new drugs is increasing. It rose by 15% from 2021 to 2022, reaching $2.3 per drug on average.
Access to big data and AI capabilities can help researchers develop new drugs more effectively and in less time. Notably, the technology helps identify the most promising drug candidates. AI can screen libraries of compounds to predict their potential therapeutic effects. As a result, algorithms generate hypotheses for creating new medicines, helping researchers find the most promising drug candidates.
Moreover, these drug candidates can be tailored to specific patients. Take cancer as an example. Not all cancer drugs are effective for all patients. Genomic databases can help find links between genomic biomarkers and drug sensitivity in cancer. This allows patients to be matched with the most effective treatments for them.
AI and big data help researchers create safe drugs for different groups of patients. AI analyzes patient data like their genetic information, medical history, and lifestyle characteristics to predict how they would respond to drugs. This makes it possible to minimize side effects.
Real-Life Example
Innoplexus has created an AI platform that makes drug discovery easier. It collects large quantities of unstructured data from research, publications, and clinical trials. AI techniques help the solution process this data. For example, computer vision classifies and extracts relevant information from PDFs and images. Thanks to ML, the platform understands the context and learns over time. All the data is inputted into one dashboard.
The tool helps companies predict the outcome of clinical trials when creating new medicines. For example, the tool predicted that Biogen’s drug for Alzheimer’s disease would fail with 70–90% certainty. It was correct: the trial did fail. This reduced Biogen’s market value by almost a third. Taking the tool’s results into consideration can help companies better manage the risks associated with failed drug trials.
Benefits
- Speeding up drug discovery
- Tailoring drugs for different groups of patients
- Developing safer medicines
- Reducing adverse effects for patients
Trends Driving AI and Big Data Applications in Healthcare
The demand for apps for precision medicine is growing fast. This can be traced back to several converging healthcare trends. The widespread availability of EHRs and genomic and other healthcare data has laid the groundwork. Coupled with this, rapid developments in AI and big data analytics made it possible to build these types of solutions. Meanwhile, the limitations and high costs of traditional healthcare methods have underscored the need for a more efficient approach. Let’s take a closer look.
The population is aging rapidly. In the US today, there are more than 54 million adults aged 65 and over (according to the US Census Bureau). This number will hit 71 million by 2030. As the population ages, the number of age-related diseases rises. These include cancer and cardiovascular disorders. Precision medicine has the potential to target and treat these diseases more effectively because it takes the unique characteristics of each patient into account. It can access data from gene sequencing, remote patient monitoring (RPM) devices, and clinical studies. This data also makes it possible to predict and diagnose diseases that are common in old age and diagnose them at earlier stages.
An increase in remote patient monitoring. RPM has become more widespread in recent years and has contributed to growing the pool of big data. With various types of wearable tech, clinicians can monitor a patient’s symptoms, vital signs, and whether or not they are taking their medication properly. AI is then used to process and analyze data generated by RPM systems to extract value and make predictions. As a result, clinicians can provide patients with data-rich medical care.
The spread of genetic testing. Genetic testing was expensive in the past, but it has become more affordable in recent years. For precision medicine, this is a positive trend, as many diseases have genetic links. Test results can identify genetic factors linked to various disorders, such as mutations. Genetic testing can also help doctors select better treatments and the right dose of medication for patients. Furthermore, screening for genetic diseases is key for preventative care.
Booming AI. The use of AI is rapidly expanding in the healthcare field. With more healthcare data available, algorithms are more commonly used. AI is helping with radiology, surgery, and vaccine research. For precision medicine, it can interpret human genomes and other biomarkers. This can help clinicians create personalized treatment plans for their patients.
Summing Up
The use of big data and AI in personalized medicine is transforming healthcare. With vast data and advanced algorithms, treatments can be tailored to each patient’s unique needs. This approach replaces the one-size-fits-all approach and makes therapies more precise and effective. It also brings opportunities for tech companies to innovate in healthcare. If you’re one of those companies and need a partner to leverage this niche, we’re here to help.
More articles on the topic
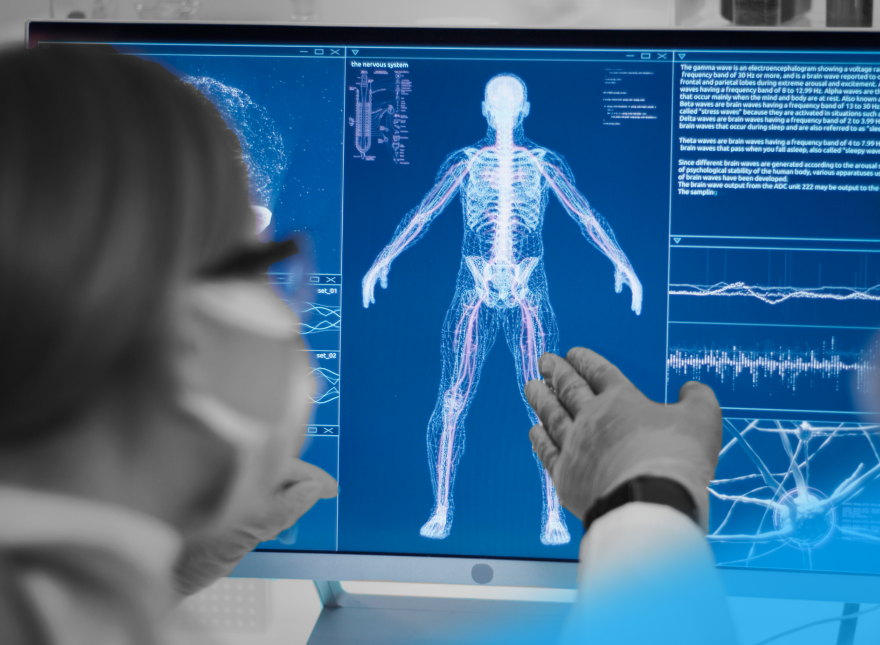
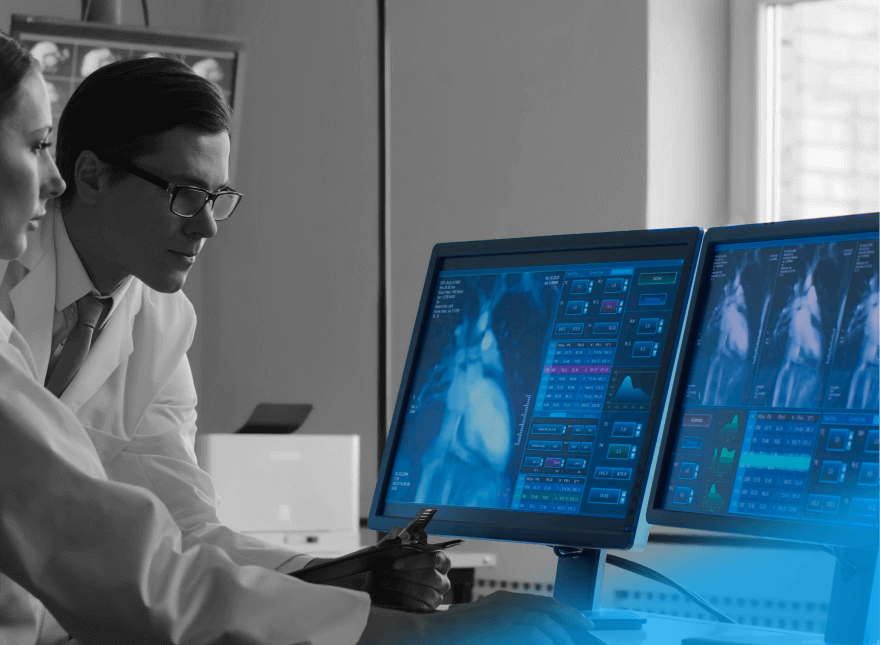
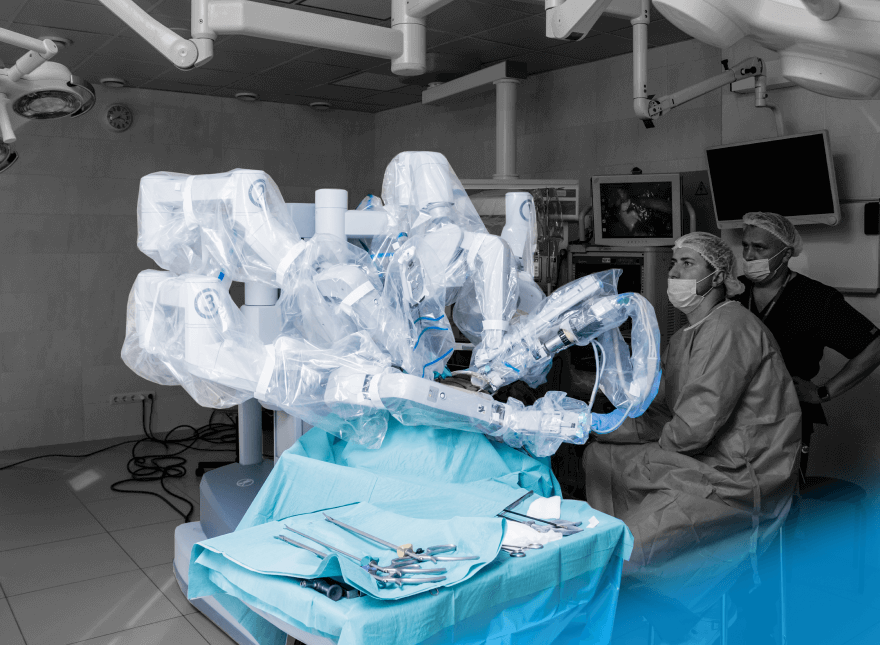
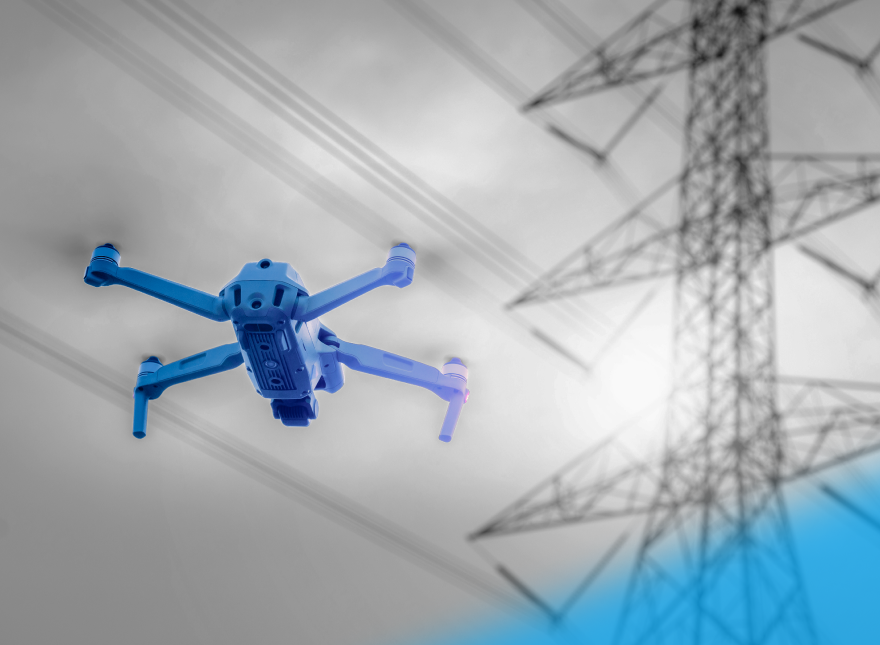
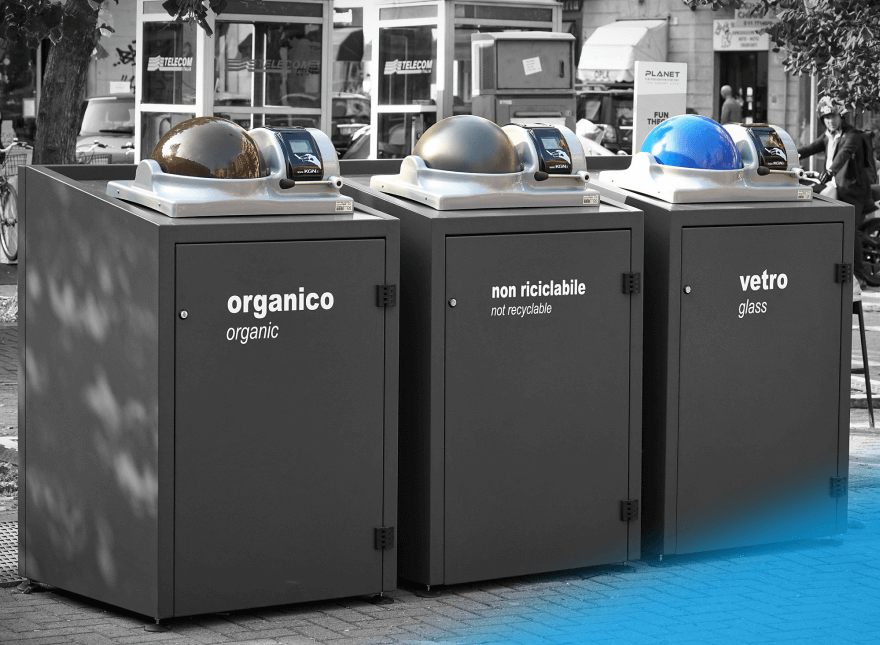
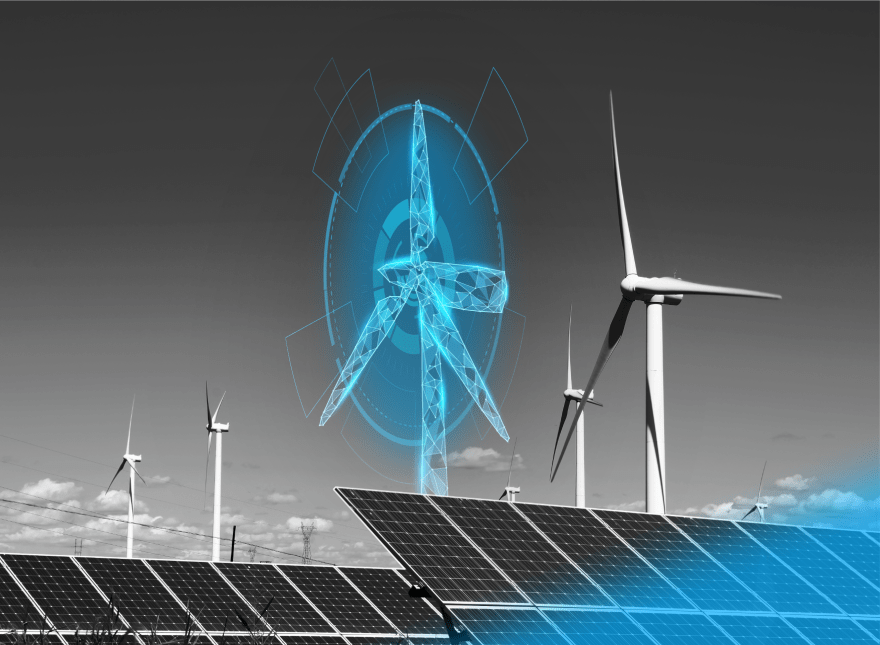