Conventional medical imaging solutions consume a lot of radiologists’ time and effort. According to this year’s radiologist survey, 90% of the respondents stated their workload increased during the past three years. Fatigued doctors are more likely to make mistakes, which hurts patients and the healthcare system, and damages the reputation of clinics. Consequently, hospital managers are looking for a way to balance doctors’ well-being and hospital spending.
IT vendors capitalize on this opportunity by developing AI solutions, which support radiologists during diagnosis. These AI-powered medical image analysis tools can identify different disorders while still in the early stages, predict the risk of cancer and neurological diseases, and allow doctors to prescribe preventive treatments. Such tools will save radiologists’ time and increase confidence in their decisions.
Global AI in the medical imaging market was valued at $21.48 billion in 2018 and is expected to catapult to $264.85 billion by 2026, registering a massive CAGR of 36.89%. Despite the growing spending and the obvious benefits, healthcare facilities have been slow in adopting AI.
This state of affairs has left people wondering how AI can contribute to radiology and why radiology centers are avoiding AI. Is it just a misunderstanding of the capabilities? Or does AI have a rather unimpressive record when it comes to diagnosis?
This article covers:
- Applications of Artificial Intelligence in medical imaging
- Obstacles along the way to widespread AI adoption
- Steps that can help overcome adoption challenges
AI Applications in Medical Imaging
“AI tools can help physicians handle a lot more data a lot more quickly and help them prioritize.”
Susan Etlinger, industry analyst at Altimeter Group
AI can be used in combination with multiple types of imaging modalities and can help with identifying abnormalities in different parts of the human body.
Screening for Breast Cancer
According to the American Cancer Society, breast cancer is the second leading cause of death among women. One in five abnormal mammograms appears to be false-positive, causing patients to suffer unnecessary distress, anxiety, and even medical interventions.
To reduce these negative outcomes, both researchers and practicing radiologists are looking to enhance the interpretation of mammograms. Here is what AI can do to help.
AI and Human Radiologists Work in Synergy
Researchers from Google Health and DeepMind cooperated with the Cancer Research Center of Northwestern University and Royal Surrey County Hospital to investigate how AI would fare in cancer detection in mammograms. The team compared the diagnosis of mammograms produced by AI to those of human radiologists. Twenty-six thousand women from the UK and 3,000 from the US participated in this study.
AI reduced false positives by 5.7% among American participants and 1.2% for British women. False negatives fell by 9.4% in the US and 2.7% in the UK. AI algorithms caught some of the cancer indicators missed by radiologists. However, radiologists also spotted cancer signs that eluded the algorithm. The results proved that it’s best if AI is used as a supporting tool for radiologists and it’s not a viable replacement for human judgment, at least for the time being.
AI-based Cancer Prediction
In another study, Regina Barzilay from the Massachusetts Institute of Technology trained AI to identify patients who are likely to develop cancer. First, she checked 89,000 mammograms against the national tumor registry. Next, she trained a Machine Learning algorithm on a subset of these images and tested the resulting model’s ability to predict cancer. The prediction was correct in 31% of the cases. This number is significant when compared to the 18% prediction accuracy of the standard Tyrer-Cuzick model used by radiologists to predict cancer.
Recognizing patients with a high risk of cancer has many benefits, such as personalizing frequent screening schedules to spot cancer in its early stages, and reducing healthcare costs, as frequent mammograms will only be offered to the women who need it.
Detecting Brain Tumors
Brain tumors, along with other nervous system cancer manifestations, is the 10th leading cause of death. Cancer.net estimates it will be accountable for taking the lives of 18,020 adults in 2020.
Using conventional methods, brain tumors take a long time to detect. The diagnosis requires a pathology lab and includes a long wait for traditional processing, staining, and image interpretation. Patients hospitalized for brain tumor surgery do not know beforehand what kind of tumor they have, which adds anxiety to the whole procedure.
Michigan Medicine addressed this problem by developing the stimulated Raman histology (SRH) technique to generate brain tissue images in less than 2.5 minutes, eliminating the usual processing steps. Researchers further enhanced this method with AI. They trained a deep convolutional neural network (CNN) on 2.5 million scans obtained through the SRH to identify ten of the most common brain tumor types. When testing the trained algorithm, the team compared its performance in identifying tumors against the pathologists judging conventionally stained and processed scans.
The CNN-based algorithm delivered 94.6% accuracy compared to 93.9% achieved using conventional histology. As in the previous example, pathologists spotted tumors misclassified by the CNN and vice versa.
“This is the first prospective trial evaluating the use of AI in the operating room. We have executed clinical translation of an AI-based workflow.”
Todd Hollon, Chief Neurosurgical resident at Michigan Medicine
By coupling traditional SRH techniques with AI, healthcare professionals significantly shorten the time needed to produce and evaluate brain images. It will improve decision making during surgery and offer expert insight to hospitals that lack trained neuropathologists.
Spotting Fractures and Musculoskeletal Injuries
Undetected fractures and musculoskeletal injuries in the long term can lead to chronic pain and a reduction in mobility. Doctors often struggle to identify relatively small fractures, especially in post-traumatic patients. Specialists are concerned with stopping internal bleeding and treating organ injuries, while small fractures can easily be missed and show their impact in the future.
Musculoskeletal Injuries
Joint motion is a critical indication while searching for musculoskeletal injuries. The traditional analysis includes anglers and de-adhesion markers, causing discomfort for both patients and physicians. One attempt to resolve this problem came from Elysium Inc., a Korean IT startup. Elysium developed a device that scans target areas on a patient’s body with 3D cameras incorporating Depth and RGB. Built-in AI algorithms work to extract and analyze data related to joint motions.
This AI-based medical imaging device can identify musculoskeletal injuries in merely five seconds of examination, increasing doctor’s productivity, and sparing patients from the inconvenience of the traditional procedure.
Fractures
Hip dysplasia is one of the hard-to-detect fractures. It affects approximately 1% of the population. If it remains unnoticed, it will develop into osteoarthritis, which requires hip replacement surgery. The good news is that hip dysplasia can be treated with a simple harness if detected within the first six months. AI can contribute largely to speeding up the diagnosis.
In the summer of 2020, MEDO.ai, an artificial intelligence startup, obtained clearance from the US Food and Drug Administration (FDA) to market its AI-based medical imaging diagnostics tool for hip dysplasia detection. This tool is the first of its kind in the world. It reviews ultrasound images and classifies hips as normal and dysplastic.
“With this innovation, MEDO gives clinicians the ability to quickly and accurately use ultrasound to diagnose hip dysplasia during routine infant check-ups.”
Dr. Dornoosh Zonoobi, co-founder and CEO of MEDO.ai
Unveiling Neurological Abnormalities
Patients diagnosed with a degenerative neurological disease will inevitably feel devastated. However, if the condition is diagnosed at early stages, there might still be a possibility to delay or even stop deterioration. In the worst-case scenario, patients will at least have time to plan their long-term care.
There are several ongoing initiatives to use AI to diagnose diseases such as Alzheimer’s and Parkinson’s during the early stages. One such effort is coming from the Octahedron project by Newcastle University in cooperation with the Newcastle NHS Foundation Trust and Sunderland Eye Infirmary.
The researchers aim to identify early signs of neurological disorders using retina OCT scans. The retina is the only part of the central nervous system that is visible without invasive procedures, and it is affected by diseases such as Alzheimer’s. High-quality retina OCT scans are available and cheap to obtain. The team trained AI algorithms to detect early signs of neurological disorders from such scans.
"The aim of the project is to use NHS data to teach computers how to detect early signs of neurological disease via retinal imaging. Ultimately, the project will help to catch those at risk earlier, before other symptoms develop."
Prof Anya Hurlbert, lead of the Octahedron project
In another example, a research team at Boston University School of Medicine developed an AI-based algorithm, which can diagnose Alzheimer’s using a brain MRI scan coupled with a cognitive impairment test, the patent’s age, and gender. Furthermore, researchers claim this algorithm can predict the risk of developing a neurological disease before its occurrence.
Identifying Diabetic Retinopathy
Diabetic retinopathy (DR) is a common complication of diabetes, and it is the leading cause of preventable blindness among adults aged 20-74 in the US. More than two in five Americans with diabetes suffer from some form of diabetic retinopathy. Despite the danger of this condition, it can’t be diagnosed by a primary care provider before vision deteriorates. Even though diabetic patients check their eyesight regularly, DR can progress tremendously before it impacts the vision.
Early detection of this condition is essential to reduce damage.
Google and Verily worked together to develop a device using AI to automatically diagnose diabetic retinopathy. The AI algorithm was tested in Aravind Eye Hospital in India, where more than 2,000 patients arrive every day. Patients simply need to look into the camera that incorporates the AI algorithm. The device is operated by a technician and doesn’t require a physician.
The drawback is that patients with cataracts can’t receive accurate diabetic retinopathy diagnosis from this AI system.
Another example of using AI medical image diagnostics systems is presented by IDx Technologies Inc. of Iowa. At the beginning of 2019, the company obtained FDA clearance for its AI-powered DR diagnostic system IDx-DR. The device uses cloud computing and AI to scan retina images and deliver results in just 20 seconds. If IDx-DR detects signs of DR, the patient is referred to an ophthalmologist for confirmation.
IDx Technologies integrated its IDx-DR tool with EHR.
“No one has ever integrated a diagnostic system where there’s no human involved. We’re ramping up slowly because we want to make sure we work out all the kinks with the EHR and the workflow.”
Michael Abramoff, founder and CEO of IDx Technologies
Why Hospitals Are Cautious about Trusting AI
AI is expected to revolutionize medical imaging. But despite the success of AI pilots and the promising results of clinical research projects, hospitals are still resisting its wide adoption.
Radiologists Are Less Than Excited about Working with AI
According to a survey conducted by McKinsey Company, the biggest barrier to adopting AI at a wider scale is radiologists themselves. They are skeptical about the diagnosing ability of AI algorithms. Another concern from the radiologists’ side is the lack of regulations governing AI adoption. For example, who will be responsible if there is a negative outcome?
In a survey of members of the European Society of Radiology, the majority wants the legal responsibility to either be shared among the involved parties or fall on the radiologist’s shoulders.
Furthermore, some radiologists are worried that if they work with AI algorithms and help to enhance them, these algorithms could potentially replace them.
Ethical Issues Surrounding Patient Data
- Data privacy and anonymization: under the EU GDPR regulation, patients have control over their data, and researchers have to seek explicit consent to use patients’ medical images to train AI. If patients agree to participate, their data needs to be fully anonymized. Another interesting question is: if a company is using patients’ data to market its AI products, are these patients entitled to financial compensation?
- Data bias: bias appears when the data used for training AI algorithms reflects only one particular cohort and doesn’t properly reflect the whole population. For example, minorities are often underrepresented, meaning radiologists will need to pay closer attention while examining people of color if hospital patients are predominantly white.
Generalizability of AI Algorithms
Algorithms obtain their “intelligence” from datasets used in training. Even if a program is performing well on data similar to the training set, when it encounters a new set of data, the performance might decline. While training, AI medical imaging companies and researchers need to keep in mind where this algorithm will be used and which population segment it will serve.
If the algorithm was developed in Europe and trained on local datasets, it will not perform the same way in African countries as diseases common in Africa will be underrepresented in the training set and unfamiliar to the algorithm.
The algorithm’s generalizability concern is amplified by the fact that most hospitals cannot assess in advance how a particular AI-powered tool will perform on their patients. Such an evaluation will require local datasets and computational infrastructure.
How to Advance AI Adoption in Medical Imaging
To ensure a bright future for AI in medical imaging, developers, researchers, radiologists, and hospital managers need to work together.
Work out Suitable Methods for Data Sharing
The performance of AI algorithms depends on the quality and diversity of training datasets. Researchers can cooperate with clinicians to prepare sets rich with annotations and metadata. If sharing datasets is not an option, it is possible to grant temporary training access while the data remains in the safety of the hospital’s firewall.
Use Comprehensive Datasets for Algorithm Training
Anyone responsible for preparing training datasets needs to be aware of the data bias and generalizability issues discussed in the previous sections. The data has to represent the population and the conditions common in the area where the algorithm will be deployed.
Another important aspect to watch is balancing data with negative and positive diagnoses. For example, if you are training AI to identify lung diseases, your dataset needs to have an equal amount of healthy lung X-rays and scans reflecting the disease you want your system to recognize.
Educate Radiologists about the Role of AI
Hospital managers need to be clear about the role they expect AI to play in their healthcare facilities. While speaking with radiologists, they have to emphasize that AI is simply another tool in the radiologist’s toolbox, not a replacement for their role.
Even devices where clinician’s interference is not required will not fully replace radiologists. They represent the first line of diagnosis, and if they spot abnormalities, humans still need to confirm them and prescribe the treatment strategy.
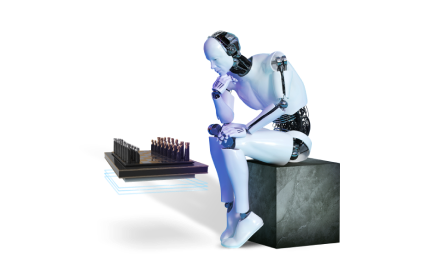
Takeaways
AI-powered image processing has many applications and benefits for the healthcare system. However, healthcare facilities are not rushing to adopt AI in their practice. It doesn’t help that some FDA-approved AI products didn’t deliver on their promises in the past. For example, in 1998, an AI-powered breast cancer screening system approved by the FDA resulted in increased annual spending without an improvement in the cancer detection rate.
Even if modern AI tools are of better quality, everyone from clinicians to companies offering machine learning radiology solutions still needs to contribute to adoption.
If you are an AI vendor, work on datasets you use to train your algorithms. Make sure they represent the conditions at your location of interest. Moreover, remember that radiologists resist using tools that they don’t understand. If your algorithm can justify its diagnostic results, doctors will be more eager to try it.
If you are a hospital manager, don’t hesitate to emphasize that AI is merely a support tool. Explain the scope of the AI application and where it stands in the diagnostics chain. Give your radiologists enough time and support to learn how to interact with the recently acquired AI solutions and understand its potential.
If you are a doctor who is considering using AI but is afraid of the consequences, remember that these tools were developed to play a supporting role. Many research experiments point out that doctors can spot disorders missed by AI algorithms. AI will speed up your work and give you the “second opinion” needed to boost confidence in your decision.
More articles on the topic
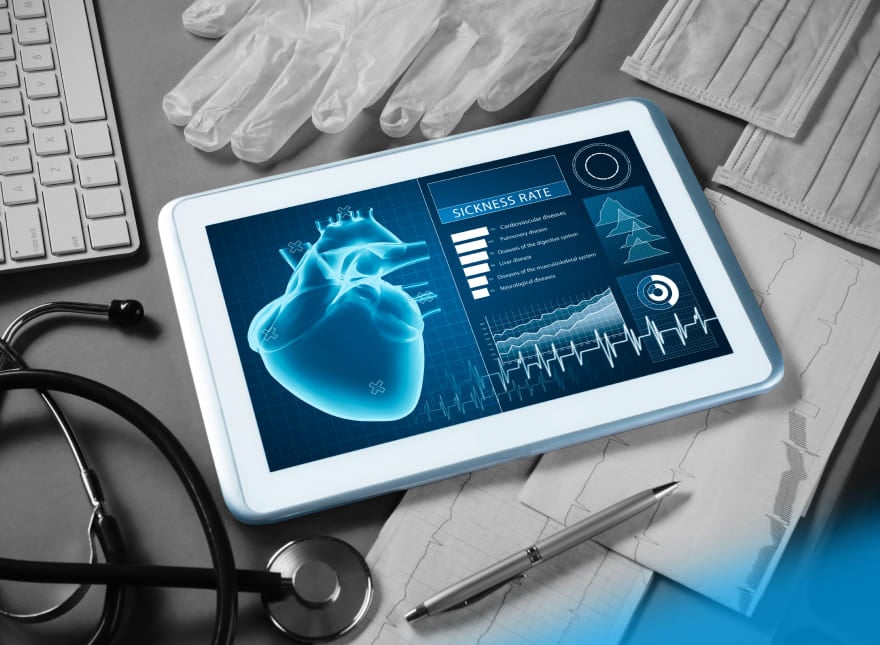
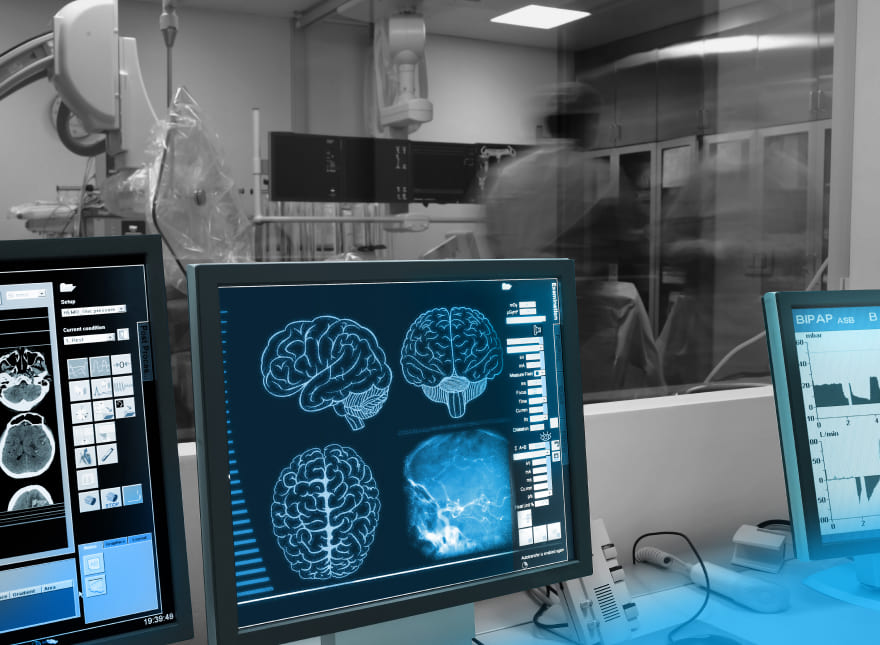
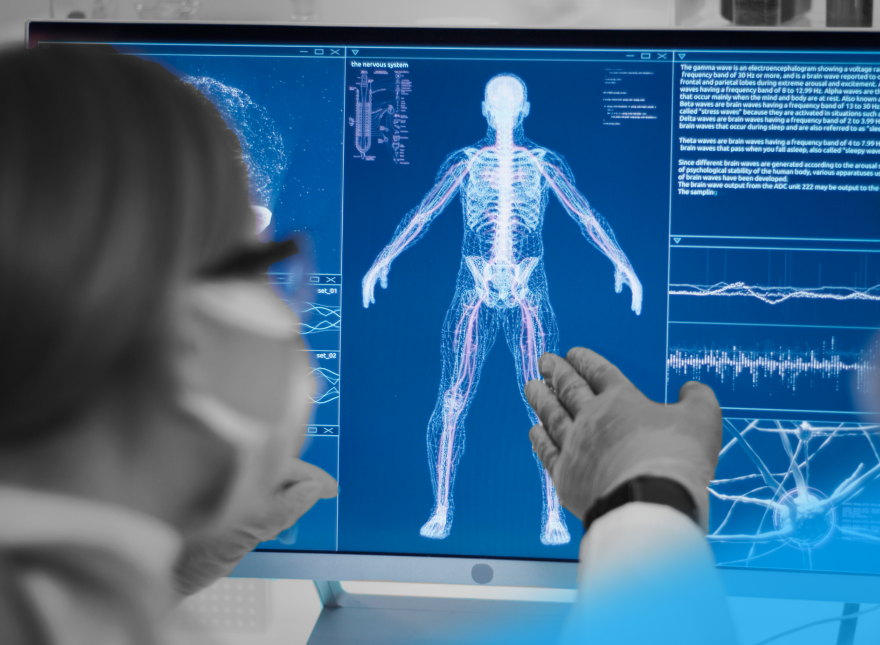
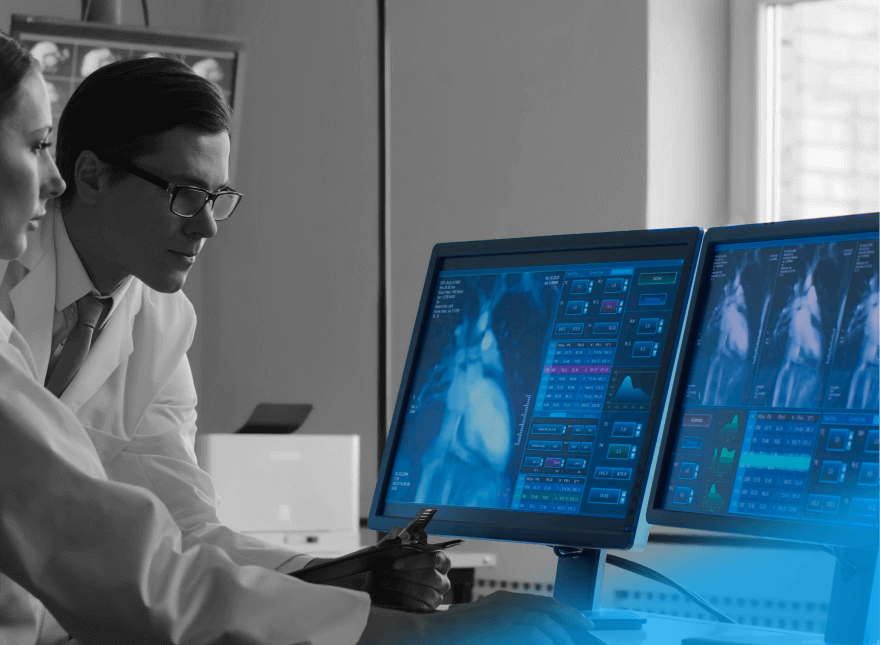
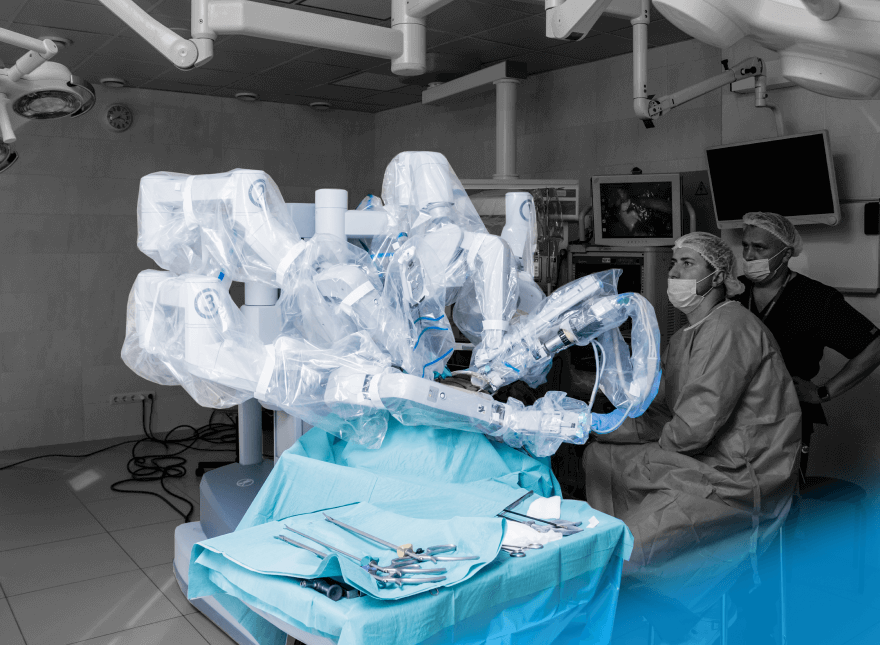
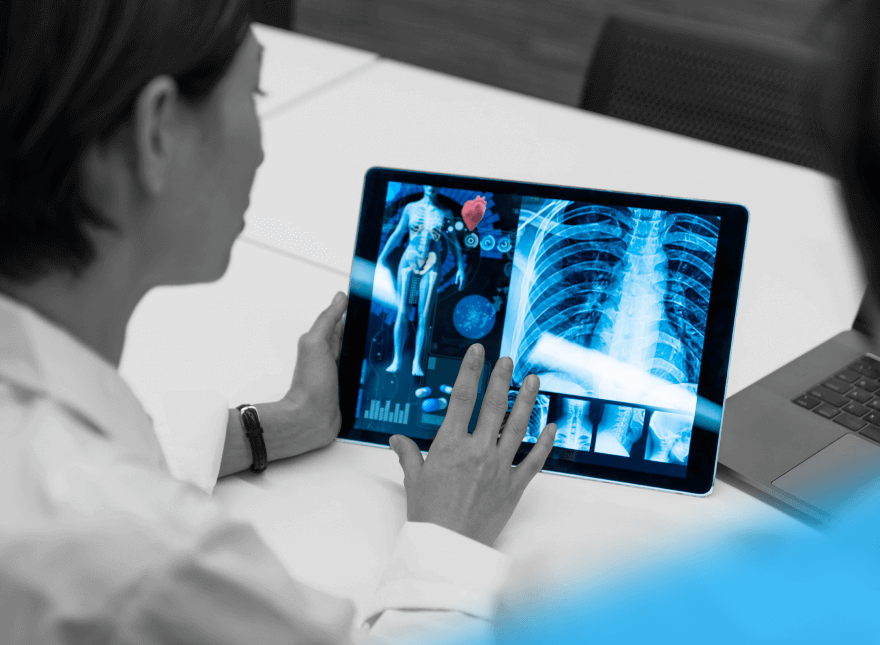